You know what I’m tired of hearing? “My email notification about recent privacy policy updates pouring from everywhere”.
Do you feel my pain?
Still, everything happens for a reason, and there’s one behind machine learning models for fraud detection (actually, there’s quite a pack of them).
Let’s find out then how using machine learning in fraud detection can benefit businesses. Ready?
First off, let’s look at the benefits of machine learning for fraud detection and challenges that trigger an increasing focus on KYC and AML.
But before we go any further, we shall look into the definition of these terms.
- KYC, or Know your customer, is a process of any business verifying the identity of its customers and evaluating potential risks of malicious intentions that might harm a company. Surprisingly enough, even reputable financial institutions fail to comply with KYC at times. Commonwealth Bank Of Australia didn’t report on suspicious activity on time and was fined for Crypto KYC failings recently.
- AML of anti-money laundering is about a variety of regulations and procedures aiming at contending fraudulent income-generating methods. Remember Bernie Madoff and his all-time largest Ponzi scheme? That’s about people like Bernie who orchestrated America’s biggest financial fraud and earned fame of “the wizard of lies stealing from people close to 65BUSD.
Back to what I was saying: there are several changes and industry challenges as a result of altering the landscape of business. Want to know a secret?
- First, increasing and more sophisticated money laundering technique, the greater focus of global regulators on AML and KYC lead to higher cost of compliance and significant risk of being non-compliant, states Everest Group
- Second, stiff competition in the financial market, fading customer loyalty and product differentiation cause particular challenges for the sales force that struggles to acquire and retain “the right customers.
And that was just the beginning.
Furthermore, as a part of AI, machine learning models for fraud detection is among top technology trends that are likely to reshape the way institutions gather, screen, and manage customer information.
But there’s one small catch.
No matter how cliché it sounds but there’s no standard solution for all the challenges on KYC and AML fronts.
Depending on business specifics, it is vital to monitor and identify the weaknesses and grow capabilities to fill in compliance gaps.
How US prudential regulators stimulate incumbents to use anti-fraud machine learning techniques
Not so long ago, the Federal Reserve System and other regulators issued a joint statement. It declares the necessity of applying innovative methods to meet Bank Secrecy Act/anti-money laundering (BSA/AML) compliance requirements.
Banks are encouraged but not obliged to enhance existing approaches to monitoring financial transactions, identifying malicious intentions, and updating clients’ risk profiles.
Amidst all the other methods, the regulatory bodies name artificial intelligence and machine learning. They state that progressive technologies are to strengthen the national financial system and protect it from illegal business activities.
Meanwhile, the bodies note that if new approaches turn to be more effective than current AML policies undertaken by banks, incumbents will undergo close monitoring aimed at identifying bottlenecks in their BSA/AML activities.
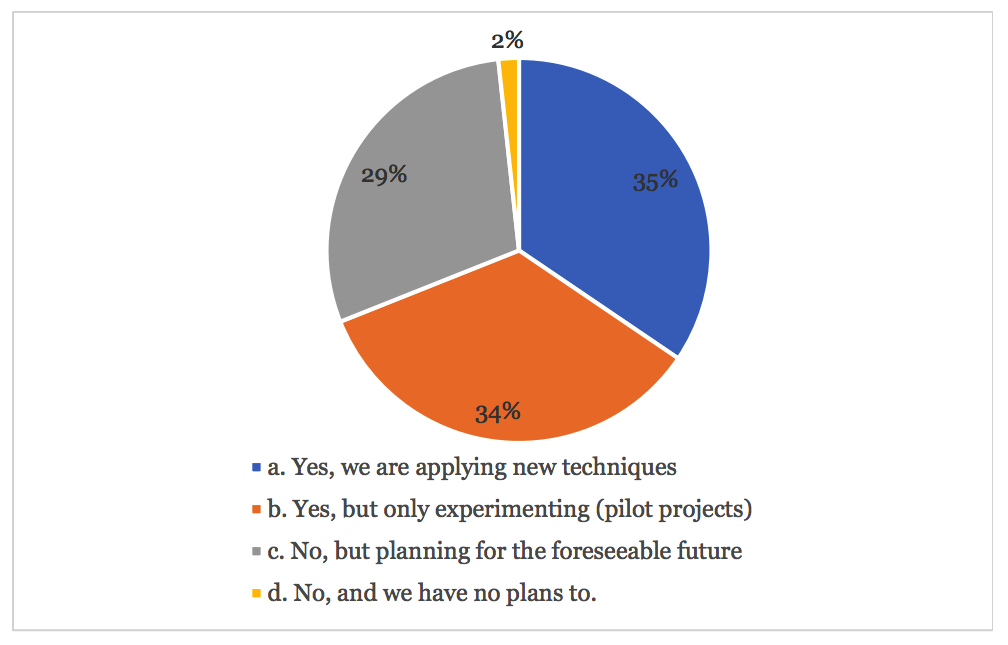
By welcoming innovations in combating money laundering and terrorist financing, the Agencies give banks the green light to deploy AI/ML.
How can banks and other financial organisations benefit from machine learning algorithms for AML?
- Cost savings and processes automation. Constant due diligence checks require sufficient human efforts while the application of machine learning results in complete automation of model development processes;
- Enormous data sets analysis. AI/ML tools can work with diverse and unstructured Big Data derived from various sources;
- False-positive statement reduction. Machine learning isn’t going to replace the old methods of AML monitoring. It can serve as an additional tool to existing programs which aim is to discover suspicious activities;
- The higher efficacy of monitoring systems. Elements of ML can be used for making decisions in cases of low and medium financial risks; complicated cases can be handled only by humans, but not without the support of AI/ML;
- Quick reaction to emerging threats. Since fraudsters are becoming more crafty, banks have to take proactive measures. With machine learning tools, they can identify usual behavioural patterns, and any abnormalities traditional methods can’t cope with.
- Better customers classification and new risk patterns. Innovations in Big Data analysis helps banks do more accurate segmentation of clients and update their risk status regularly. What’s more, with smart technologies, it’s easy to discover real patterns different from written rules.
What you will learn:
Where to start with fraud detection and machine learning?
To begin with, let us assure you that the key to success here is to try and test the waters with different machine learning techniques to detect fraud.
Then, you can weigh all the benefits and identify the most suitable system among machine learning models for fraud detection at hand.
They say the chances for favourable outcome increase when you joggled both unsupervised and supervised machine learning-based fraud detection mechanisms combining the two helping business to tackle compliance challenges.
Why?
Because the ability of any financial institution to verify the info customers submit is essential.
Let’s not forget the story of Deutsche Bank. A year ago was fined by FCA (one of the British regulators) and cost 204MUSD: it happened because the German lender had anti-money laundering controls in place that were not reliable enough.
But you’re probably wondering about a winning technique that can help your company with fraud prevention.
While there’s no universal solution, the problem list any business might face is similar.
Decide what your gaps are and see where you can improve when using machine learning for fraud detection:
Operating lean data from multiple sources
This method helps companies with a unified pool of data: an intelligent algorithm that powers machine learning and fraud detection gathers data quickly and at a lower cost.
Tons of records from internal and external sources are pulled all together in one vault with further consolidation, analysis, and classification.
In other words, machine learning-based fraud detection is a solution to search challenges for it encourages MDM (short for Master Data Management) from one single view that unites “noisy and often incoherent records.
Therefore, machine learning techniques to detect fraud help enterprises gather critical data under one roof glueing separate pieces of it together.
Building strong prediction capability in the long run
The potential of machine learning and fraud detection to get smarter after some time is another appealing feature of this modern method.
Intelligent data mining became real with ongoing updates and past cases processed by ML and used to predict possible fraudulent transaction that is likely to happen.
Have you ever found yourself how it would be to have a crystal ball and be able to foresee the challenges on the way?
What’s more, by fine-tuning the knowledge, machine learning-based fraud detection is a model that incorporates the lessons learnt into future search and analysis.
Beyond that, layers of new information can be added on top: tagging, for instance, marking a particular transaction or a purchase coming from a recently classified unstable location as suspicious.
Choosing patterns instead of rules to verify a transaction
Just imagine that a bank’s customer wiring the money to his sister abroad.
The sum he is transferring is somehow larger than a typical amount for such a transaction. Further, his sister’s husband goes to a bank and takes the funds.
Now consider it this way: the relations among all the participants are not that clear for a bank, e.g. mismatching last names etc.
Thus, a rule-based tool is unlikely to mark this transaction as suspicious.
By contrast, a pattern-based method would do a much better job recognising the catch if a similar thing occurred frequently; machine learning models for fraud detection signal yellow (not yet red though) light of possible money laundering requiring a further look into.
Reducing human error: battling insecurities
Augmenting human intellect with machine learning techniques to detect fraud is essential for today you cannot automate certain aspects of KYC.
Therefore, certain areas of inspection are still carried out manually. Although an algorithm is smart enough to address some compliance issues, a necessity for human touch is hard to deny.
After all, fraud attempts in, e.g. the financial sector is far more complicated than other verticals: banking is where scammers get very crafty.
That’s why leaving machine learning-based fraud detection unattended could lower the chances for spotting money laundering schemes and mitigating the risk promptly.
Screening for a broader range of risks
How can machine learning prevent fraud with a variety of new risks popping up each day?
Often called the new frontier in anti-money laundering, the ML-powered system can cover lager quantity of risk signals.
Put it another way: a model would be capable of identifying the transaction to some emerging Hezbollah that might be already making the headlines but absent in official blacklists.
To top it off, machine learning models for fraud detection can adopt context-driven identity matching that is superior to conventional ‘name match’ technique of rules-based systems that exist today.
And just think: the difference is in a more significant number of parameters to account for to get holistic about the analysis of factors and identify patterns with greater accuracy.
Onboarding customers: keeping it all in sync
Again, when it comes to rules-based onboarding tool, you’re relying on a static and off-the-shelf solution. But that’s not all the technology has to offer today.
But here’s the exciting thing: using machine learning in fraud detection has multi-fold benefits with dynamic questionnaires being one of them. Hello, IPA capabilities and its perks for a business!
A feature that can adapt sets of questions as per earlier responses of a client, intelligent process automation is considered to be at the core of the innovative operating model.
Additionally, IPA capabilities can do the magic and KYC profile update backlogs of any financial institution.
Similarly, it can improve the detection of unusual KYC based on transaction type in real-time such as a client from Gibraltar purchasing hundreds of Canada goose parkas.
Online fraud detection using machine learning: UOB use case
Good news is that more and more banks are deploying machine learning to combat fraudulent actors.
The alliance of United Overseas Bank (UOB), Tookitaki and Deloitte is an excellent example of augmenting anti-money laundering campaign with new cutting-edge technologies.
The Anti-Money Laundering Suite (AMLS ) developed for UOB was geared towards reducing costs on AML activities, increasing the efficiency and simplifying these processes.
Main results achieved within the AMLS project:
- 5% increase in true positives and a 40% drop in false positives;
- 60% reduction in false positives for individual names and 50% reduction in false positives for corporate names;
- 40% increase in operational efficiency.
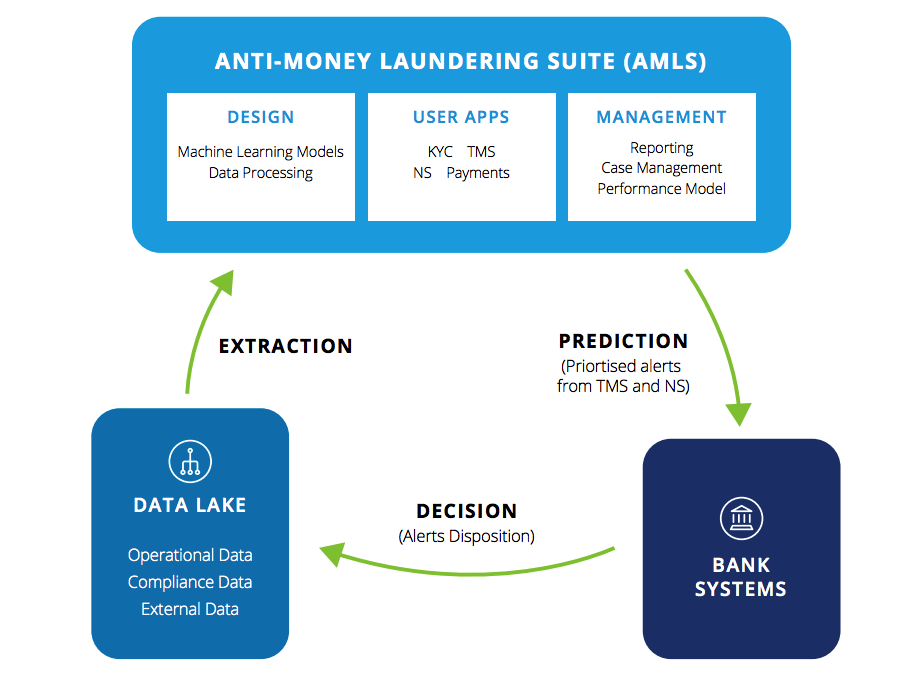
Why isn’t machine learning applied for AML and fraud detection everywhere in the FinTech?
Global collaboration. Banks can’t generate Big Data by themselves, for this purpose, they need to collaborate with information agencies and each other and build a global data-sharing network. Currently, the lack of customers’ information prevents financial institutions from deploying machine learning.
Lack of experts. Since very few people have solid knowledge in both financial services and AI/ML, solutions developed by startups don’t always meet the established goal.
Explainability. Machine learning has the neural nets method at its core. Despite the ability for delivering accurate results through self-education and forecasting, the outcome is often tough to interpret.
The need for supervision. To get the desired outcome, especially in the case of detecting terrorist financing schemes, models should be taught correctly by an expert.
Integrating automated KYC verification solutions is a big deal, but we at JustCoded know the ropes.
CapitalRise
CapitalRise, a fundraising platform for property investments, is one of our clients.
To handpick only the best proposals, CapitalRise carries out rigorous underwriting and due diligence processes.
The company provides investors with details about a property developer, its financial state, history, background, credit story, etc.
We’ve integrated a KYC solution provided as part of Goji Investments services which enables online verification and streamlines the process in case additional documents are required.
Shojin
Shojin is a UK-based property co-investing and equity fundraising platform.
On their official website, the company states that each project undergoes three stringent stages of the due diligence procedure.
Location, market estimation and a financial appraisal are taken into account within the first stage of a borrower’s review.
If a project passes this stage, experts have a closer look at other aspects of a proposal – a seed capital, duration, financial plan, expenditures.
The platform has automated KYC and AML checks run through MangoPay.
So what does all this mean?
The KYC and AML regulations can be harsh if you employ a wrong technique to deal with evolving legislation.
However, It’s your decision and your business that you need to keep afloat.
The choice is yours.
With so many tools and machine learning models for fraud detection, it would have been a crime not to harness the potential of this modern technology to help you scale and stay compliant.
It’s easier than you think.
At JustCoded, we work with dozens of clients creating finnovative solutions and empowering change while keeping compliance issues at bay.