The amount of data used by financial middlemen is increasing by leaps and bounds.
Nowadays, the Big Data Analytics widely applied in the banking practice and used for finance can hardly surprise anyone who is well aware of the topic.
More and more players start seeking far more innovative technologies to solve problems connected with data processing and analysis.
As a result, artificial intelligence (AI) and machine learning (ML) successfully applied in computer science and other spheres in the past have now become a new trend in financial technology solutions.
Artificial Intelligence is a scientific approach implying that machines perform complicated tasks by mimicking the cognitive activity of humans.
It is about modelling such functions of human minds as “learning, “problem-solving and “decision-making.
According to Wikipedia, machine learning is an array of AI methods aimed at tackling numerous similar tasks by self-learning.
ML methods include multiple statistical tools, such as Big Data Analysis, neural networks, expert systems, clusterisation etc.
The variety of these means help to process data faster and more effectively.
Even though machine learning requires enormous computational powers and out-of-the-box specialists, the number of perks it promises to the financial industry is impressive.
What you will learn:
Artificial Intelligence and machine learning in finance
It’s worth mentioning that only a number of automated business processes in banking and finance have AI and ML as their core.
Though automation is a compulsory part of the financial intermediaries’ activity, it is rarely capable of coping with complex tasks.
Deep learning, on the contrary, is doing this just fine.
How exactly does it work?
The learning ability is powered by a system of algorithms being able to derive information and build patterns out of the amount of data being studied.
The complex algorithms used in the everyday routine of financial institutions are expected to ease their operations significantly.
Among them are financial monitoring, customer support, risk management and decision-making.
Hypothetically, the time for smart machines to replace workers in most of those as mentioned earlier and other business processes is just around the corner.
Why is applying machine learning so seductive for a growing number of financial institutions? Let’s see.
Fraudulent activity prevention
KYC and AML checks are an integral part of any financial operation.
These abbreviations stand for Know Your Customer and Anti Money Laundering.
These policies focus on banning suspicious operations and preventing criminal activity. According to the Coalition Against Insurance Fraud Report, insurance companies lose $80 billion annually due to fraudulent activity in the insurance market.
The solutions of machine learning are geared towards building models for identifying questionable operations based on the analysis of the transactions history.
Moreover, the technologies of machine learning are extensively used for biometric customer authentication.
As security precautions have always been of the utmost value in the financial world, the development of such authentication methods acquires greater importance.
There are a lot of examples of FinTech startups implementing the know-how of a popular Apple Face ID technology designed for authorisation through face recognition technology.
MasterCard uses facial recognition for payment procedures and VixVerify for opening a new current account.
PayPal, for instance, is going to move further and elaborate silicone chips that can be integrated into a human body.
And that is not a full list of ideas which soon will become a usual thing. FinTech continues to stun.
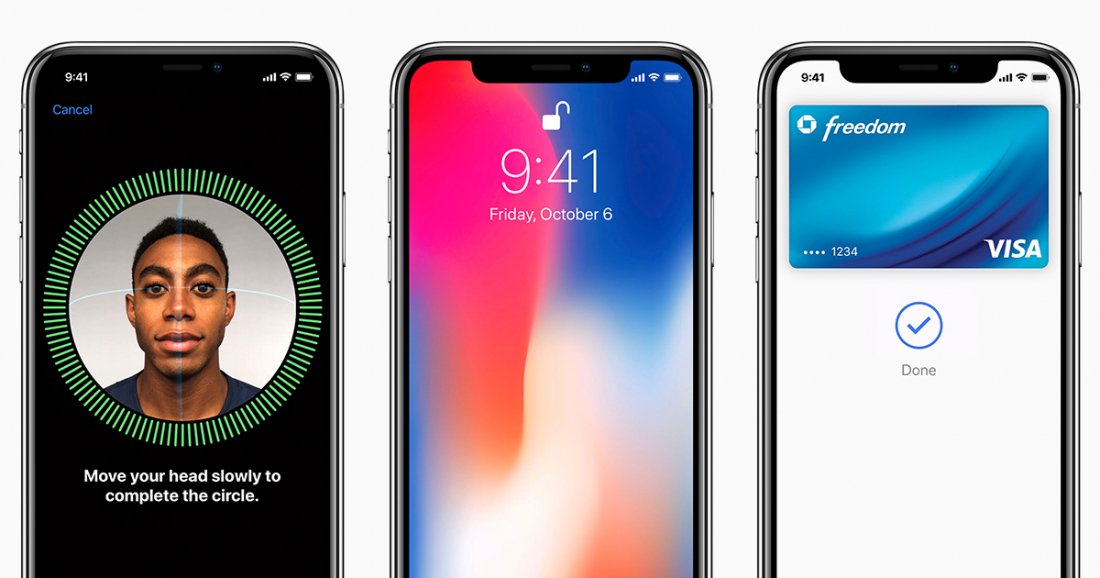
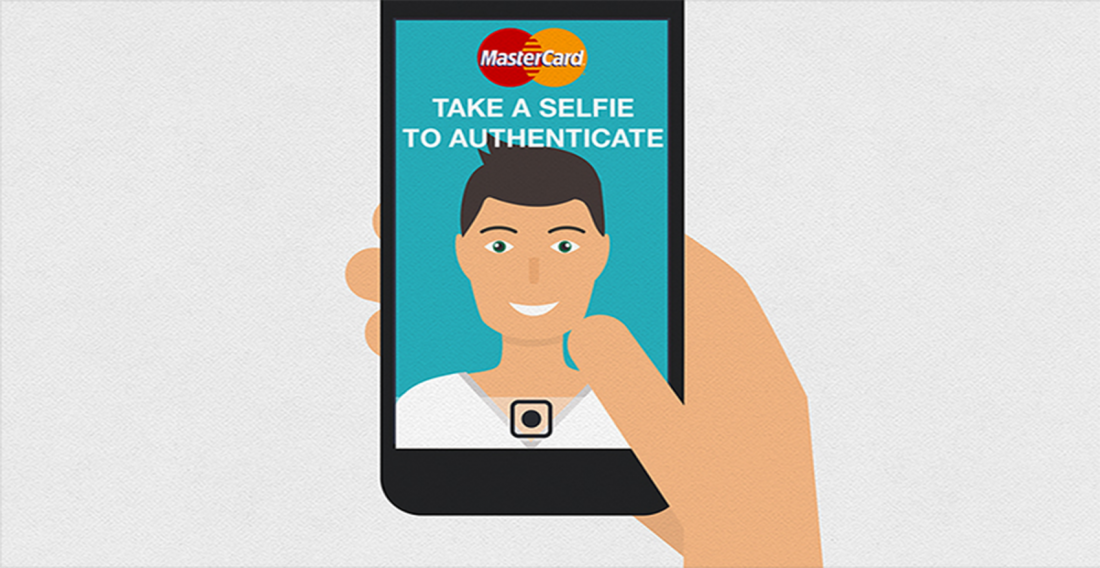
Investment gains prediction
“Am I going to benefit or lose from this investment? is the question keeping investors awake at night.
Indeed, one can hardly be 100% sure about what the future holds for them.
Fortunately, machine learning algorithms are going to become indispensable helpers and real fortune tellers in this deal.
Established financial agencies and brand-new FinTech startups have recently started creating their programs and packages for algorithmic trading built with various programming languages such as Python and C++, in particular.
Machine learning provides powerful tools to investigate the patterns of the market.
Each computational task can be carried out with the help of a particular algorithm, e.g. linear regression, decision trees, cluster analysis, etc.
The implementation of these methods has enabled traders to determine the most probable outcome of their strategy, make a trading forecast and choose a behavioural pattern.
Binatix was one of the first trading firms to use deep learning technologies.
The company employs AI-based methods to spot investment opportunities; without them, it would still be a game of random chance.
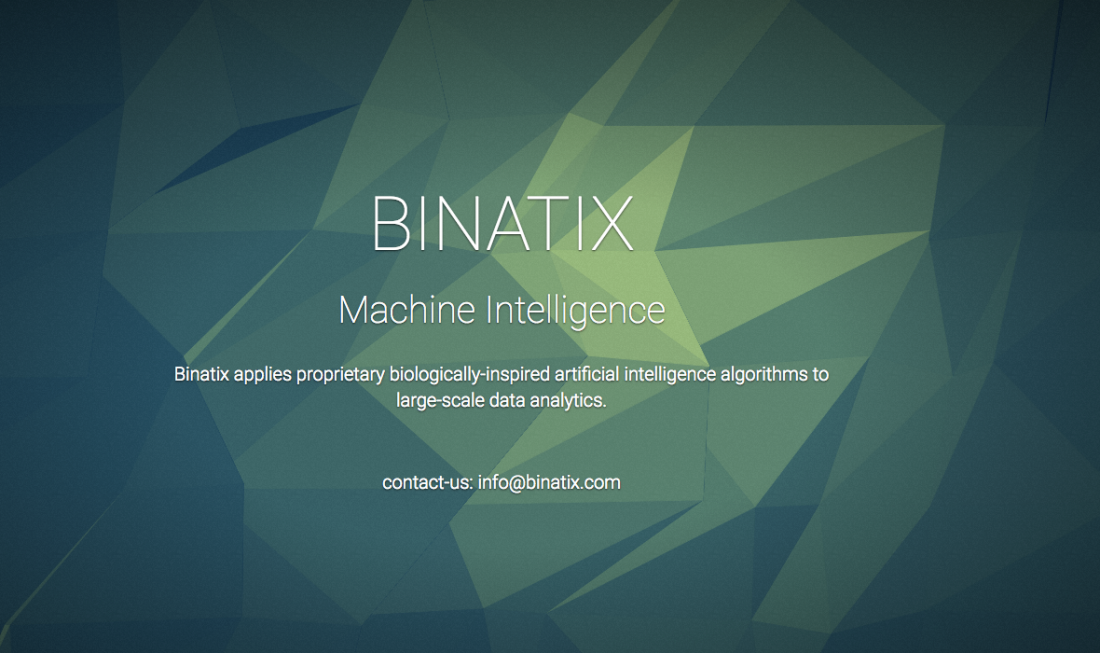
Advanced digital helpers
Another indisputable advantage of using machine learning in financial services is the invention of smart personal advisors and chatbots.
The client always values being addressed carefully and with the right attitude.
The new generation of digital helpers has allowed banks to leverage clients’ satisfaction and loyalty significantly.
Financial companies hire tech-savvy specialists to develop robo-assistants that can give advice and make recommendations according to the spending habits of customers.
Greater use of chatbots helps clients to get assistance far quicker rather than to wait until a human gains insight into the situation.
The world is already overwhelmed by personal secretaries as Apple’s Siri or Google Assistant.
FinTech companies are also on the path of creating digital helpers that won’t give way to popular toys.
In some cases, it’s pretty hard to understand who you are being serviced by either a real person following the instructions or a chatbot.
The potential of AI and Machine Learning in the banking industry
AI-based technologies have empowered computers to handle new information, compare it with existing data more efficiently, examine market trends more accurately and make more realistic predictions.
It enables financial institutions to make well-informed decisions.
No wonder that this opportunity continues to attract the attention of more and more large banks entering the FinTech industry.
For instance, in the US using super-smart technologies for anti-money laundering is welcomed by regulatory authorities who have a firm hand over the banking industry and financial market.
In the Joint Statement on Innovative Efforts to Combat Money Laundering and Terrorist Financing, the SEC and other financial regulators call on banks to implement ML/AI elements in their existing monitoring systems to protect the financial system from suspicious and fraudulent activities.
Let’s take a look at the applications of machine learning for the benefit of a bank.
Credit risk management
Assessing and forecasting debtors’ creditworthiness is quite a headache for most of the banks.
Machine Learning is believed to be a real tidbit in this tricky business.
Many debt lending companies have long been successfully working with ML algorithms to determine the rating of borrowers.
Among them is Kabbage, a platform for small business investing, LendUp specialising in micro-lending and Lending Club, a strong player of the FinTech market.
Unlike conventional ways of evaluating clients’ creditworthiness, machine learning provides a more in-depth and better analysis of clients’ activity.
ML algorithms help analyse possible changes in a client’s status and provide a dynamic assessment of their lending capacity.
In such a way, risk managers can identify borrowers with rogue intentions and protect their companies from unfavourable scenarios.
High-Quality client service
The times when bank customers obediently waited in lines are gone.
Today everyone wants to be provided with top-class services in the right place and at the right time.
AI and ML techniques have considerably contributed to language processing, voice-recognition and virtual interaction with customers. A
s a result, most of the basic inquiries received from the clientele can be answered by chatbots, whereas serious requests still need to be addressed by real people.
It helps cut overall expenses and improve the quality of customer support.
Some large banks have already begun testing out the ability of their robo-helpers to interact with customers. Who knows, maybe, they will entirely replace human managers in the years to come.
Custom marketing
This advantage of machine learning may not seem obvious to you. However, deep learning is indeed just ideal to meet marketing goals.
We’ve already mentioned that algorithms are quite useful when it comes to predictions and, therefore, marketing forecasts.
By analysing the previous reaction of bank customers to marketing campaigns, their interest in bank products and usage of financial apps institutions can create custom marketing strategies and boost their sales.
Thanks to high-performance algorithms, banks are now able to perform instantaneous analysis of the data from social nets and other web sources and convert it into information useful for practical marketing goals.
So, we can surely say that both AI and ML in bank marketing are going to become the next hot trend and turn the entire industry upside down.
Cybersecurity
Cyber attacks are the scourge of any online business, and FinTech startups are not the exception.
No matter how safe and secure your financial advisor is, there is always a risk of security breaches occurring.
Machine learning capabilities of detecting and tracking suspicious activity are vitally crucial for decreasing the probability of cyberattacks.
Non-AI tools used for security maintenance appeared to be less efficient comparing to more advanced tools.
Sophisticated security systems are pricey and not so easy to build, that’s why most of the banks are still hesitating to change them.
The possible way out of this situation might be partial re-building of the existing systems or integrating some elements of AI and ML into them.
How is machine learning used in finance: best practices
Here’s a squad of pioneers who have reaped the benefits of machine learning in banking and are currently demonstrating positive results.
Bank of America and its virtual financial assistant Erica
Erica is a virtual helper built in the Bank of America mobile application.
Interaction with Erica is possible by voice or messages depending on users’ preferences.
The assistant helps mobile users with different things such as checking account balances, paying bills, making transactions or searching for the necessary info.
Erica self-trains using its conversations with the bank’s clients. The development team supporting Eruca is continuously upgrading its features.
Now, the bot is capable of notifying clients about reaching preferred rewards status.
The UOB and its Anti-Money Laundering Suite (AMLS)
The project group consisting of the UOB, Deloitte and the Singapore-based RegTech startup, Tookitaki, has developed a solution for augmenting the bank’s anti-money-laundering system.
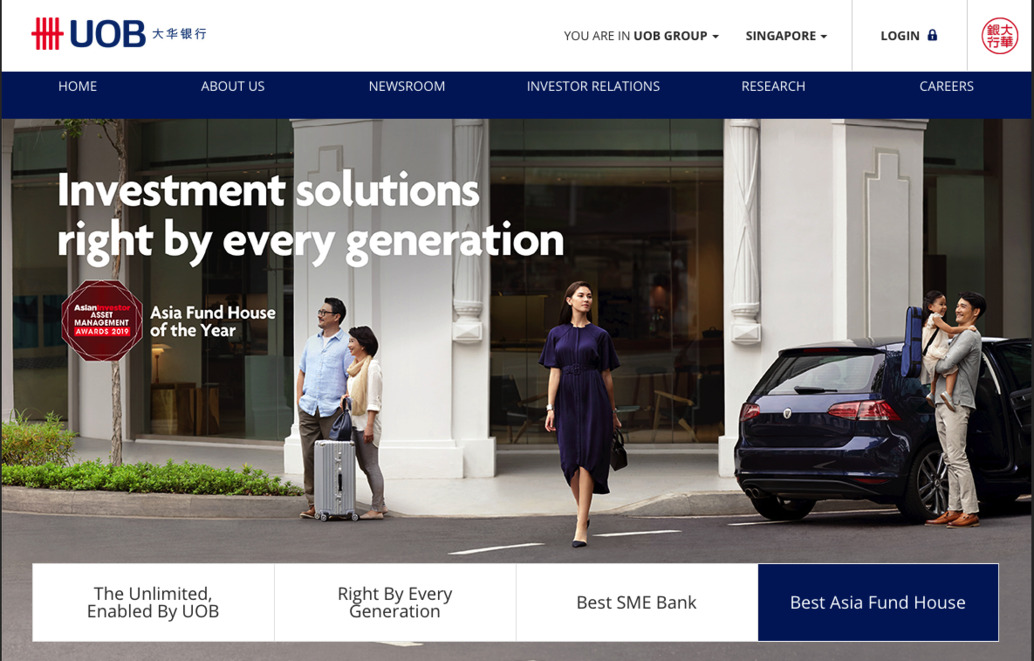
Initially, it was a ‘sandbox’ version, but then the AMLS was put into production. The platform based on machine learning technologies is used for KYC procedures, payments and transactions monitoring, name screening, etc.
The outcomes of the project were: lower administrative costs, better efficiency, more straightforward AML/KYC compliance procedures.
Manulife and its smart underwriting
It’s a great example of machine learning applied to finance and insurance.
Manulife, a leading Canadian insurance company, has launched a Manulife Par to provide life insurance underwriting services based AI algorithms.
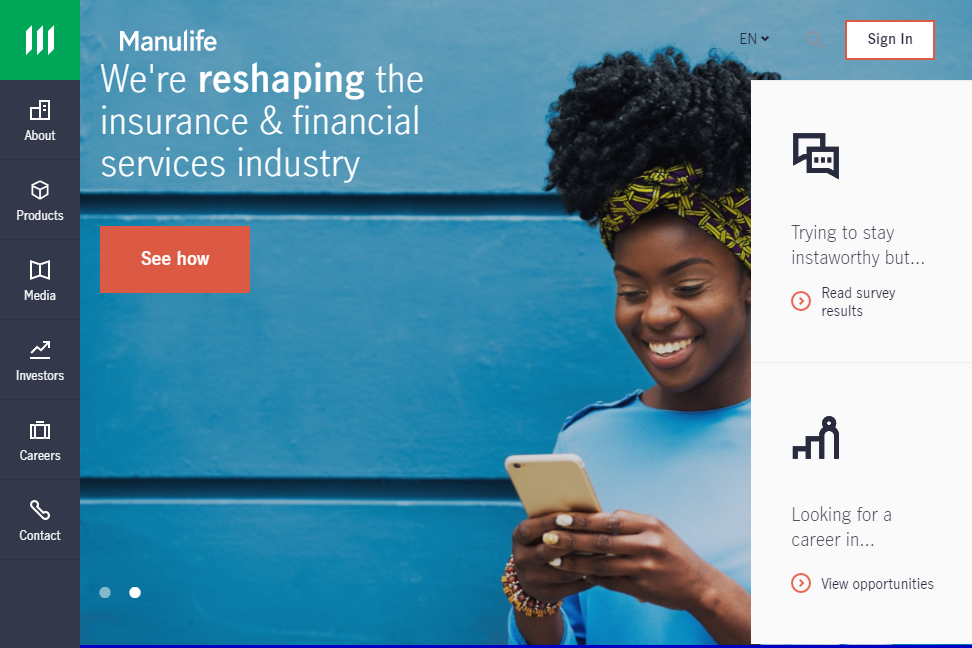
The overall goal of the innovation is to simplify the process of clients’ buying insurance, make it more appealing to people through discounts and rewards schemes.
Manulife hopes to increase the efficiency of the underwriting process by reducing unnecessary cycles of work.
Wealthfront and its automated advisors
Wealthfront kicked off the automated advisory project with AI at its core long ago when others were contemplating this idea.
The primary role of AI in financial advisory services is to deliver a personalised experience to customers.
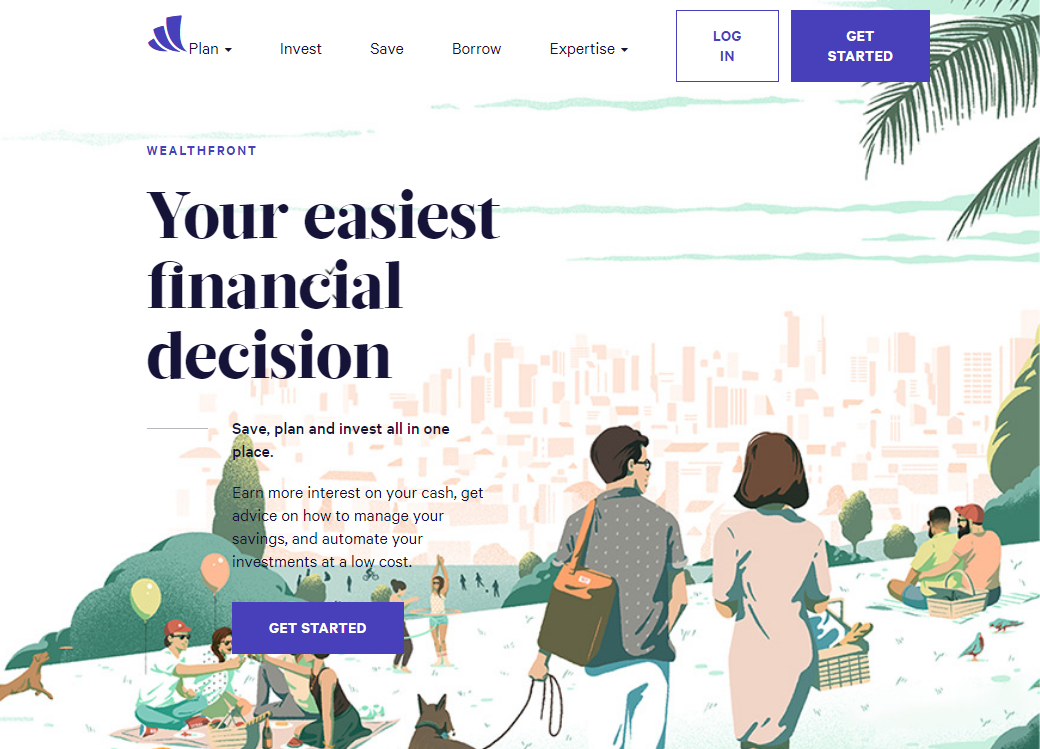
The Wealthfront’s AI solution can track users’ financial activities and provide recommendations on the best investment options in terms of fees, tax losses and cash drags according to people’s behavioural patterns.
Even though the solution is oriented mainly to Millenials who are big fans of advanced technologies, the company doesn’t eliminate the human role in advisory services.
JP Morgan and its COIN program
The largest American bank, JP Morgan, has paired machine learning and fintech for its internal project aimed at automating law processes.
A new program called COIN is to automate documents reviews for a chosen type of contracts.
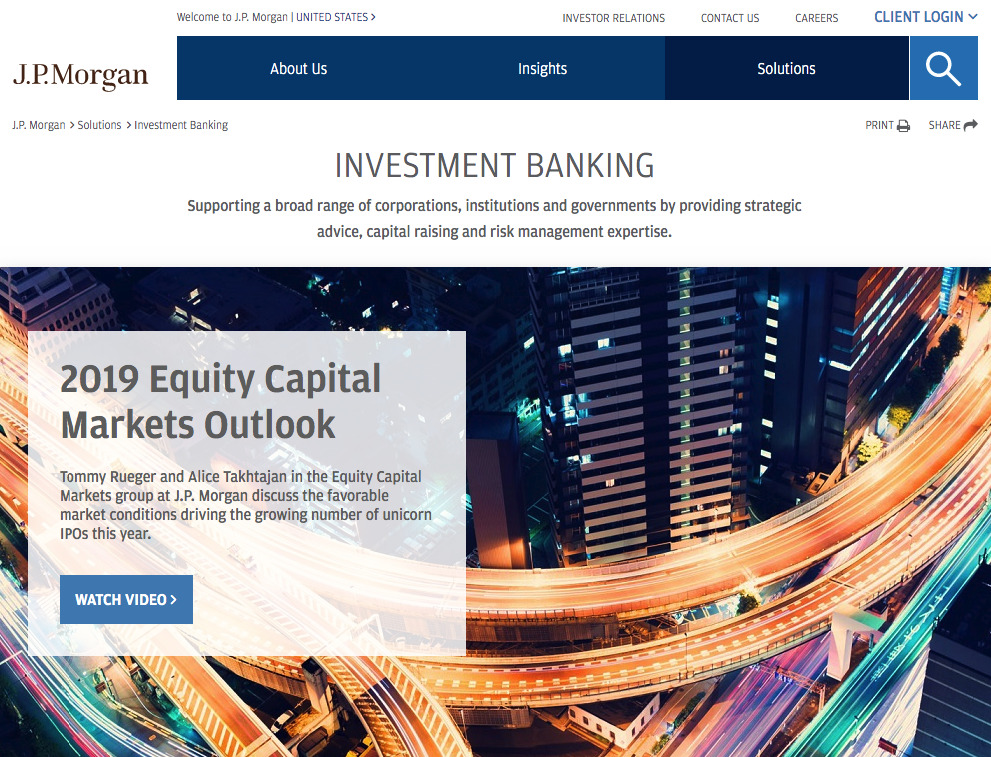
The algorithm works as follows: it analyses data from banks’ contracts, learns, identifies and groups repeated clauses.
It’s incredible, but the software does the job in a few seconds, which required 360,000 working hours before.
The results of the COIN program are better accuracy in the contracts reviewing and reduced administrative costs.
Fintech and Machine Learning: the outcome
Nothing is perfect in the world, and even machine learning has its limitations.
Humans control automated systems and losing control is quite dangerous.
Continuous hucker attacks on social accounts together with fake news heat the situation that often leads to irreversible consequences.
Constant security support requires considerable human resources and great technical facilities; that’s why some financial institutions disregard it.
As a result, terabytes of personal info are stolen every day.
Even chatbots tend to misbehave (that happens quite frequently) and drive customers crazy who, consequently, demand human assistance.
All in all, ML applications in finance have contributed to positive changes in the FinTech industry by offering feasible solutions for data analysis and decision-making.
Integration of the elements of deep learning can solve plenty of tasks in FinTech.
Advanced technologies of machine learning in banking and finance are going to lead the industry towards better relationships with clients, lower operations costs and higher profits soon.
However, the industry is still far away from being ruled by non-human creatures.